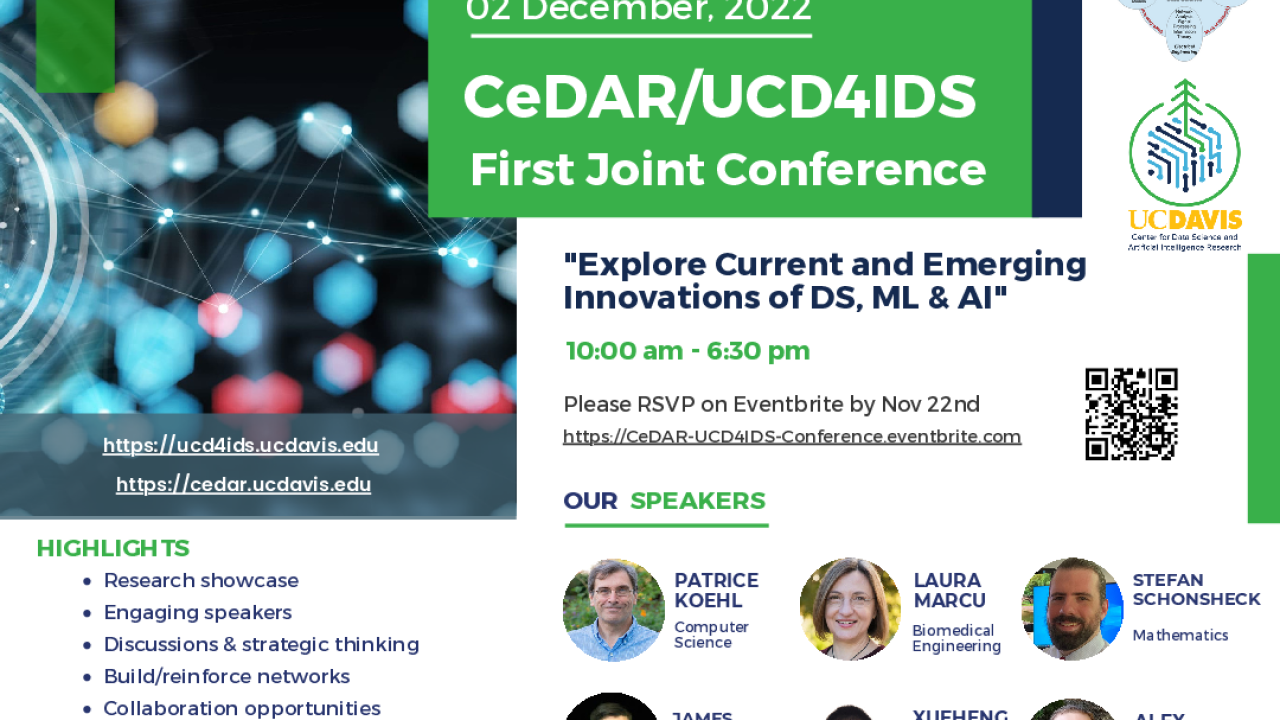
Event Date
Dear Colleagues and Graduate Students,
CeDAR (Center for Data science and Artificial intelligence Research) and UCD4IDS (UC Davis TETRAPODS Institute for Data Science) will hold our first joint conference on Friday, December 2 at 1147 Math. Sci. Bldg. starting at
10am.
The details are below, but if you plan to attend this conference (even partially), please visit https://CeDAR-UCD4IDS-Conference.eventbrite.com and register for it by Tuesday, November 22 because we need to count the number of people who want lunch and who plan to attend our reception.
Synopsis: CeDAR and UCD4IDS were both established three years ago with the support from the UC Davis Office of Research and the National Science Foundation, respectively, to advance research and education focused on data science in our campus. Despite the tough times due to the COVID restrictions, both have thrived: promoted many interdisciplinary collaborative projects, generated many publications, disseminated our research results through conferences, seminar talks, and open software packages, and were instrumental in raising substantial amounts of grant money. We have now reached a point to have our first joint conference in the in-person format to showcase the research of some members of CeDAR and UCD4IDS and provide more discussions and opportunities for further collaborations among different disciplines. This event will involve faculty members, postdocs, and graduate students.
Schedule for Friday, Dec. 2, 2022 @ 1147 MSB
10:00-10:10: Introduction/updates
10:10-10:55: Talk 1 Patrice Koehl (CS)
11:00-11:45: Talk 2 Alex Wein (Math)
11:45-13:15: Lunch
13:15-14:00: Talk 3 Laura Marcu (BME)
14:05-14:50: Talk 4 Xueheng Shi (Stat -> Univ. Nebraska)
14:50-15:05: Coffee Break
15:05-15:50: Talk 5 Stefan Schonsheck (Math)
15:55-16:40: Talk 6 James Sharpnack (Stat -> Amazon)
16:40-16:55: Summary / Discussions
17:00-18:30: Reception
======================Title & Abstract==========================
Patrice Koehl (CS)
Title: A Physicist's View on Partial 3D Shape Comparison
Abstract: Scientists have access to a wide range of digital sensors that allow them to report at multiple time and length scales on the subjects of their studies. Finding efficient algorithms to describe and compare the shapes included in those reports has become a central problem in data science. Those algorithms have gained from developments in computational geometry and in machine learning. In this talk I will present another source of support to further improve those algorithms. Using techniques from statistical physics, I show that we can define a possibly partial correspondence between 3D shapes, with a cost associated with this correspondence that serves as a measure of the similarity of the shapes. I will illustrate the effectiveness of this approach on synthetic data as well as on real anatomical data.
Alex Wein (Math)
Title: Computational Complexity of Tensor Decomposition
Abstract: Tensor decomposition is an important subroutine in numerous algorithms for data analysis, including topic modeling, community detection, clustering, and dictionary learning. We consider a simple model for tensor decomposition: suppose we are given a random rank-r order-3 tensor---that is, an n-by-n-by-n array of numbers that is the sum of r random rank-1 terms---and our goal is to recover the individual rank-1 terms. In principle this decomposition task is possible when r < cn^2 for a constant c, but all known polynomial-time algorithms require r << n^{3/2}. Is this a fundamental barrier for efficient algorithms? In recent years, the computational complexity of various high-dimensional statistical tasks has been resolved in restricted-but-powerful models of computation such as statistical queries, sum-of-squares, or low-degree polynomials. However, tensor decomposition has remained elusive, largely because its hardness is not explained by a "planted versus null" testing problem. We show the first formal hardness for average-case tensor decomposition: when r >> n^{3/2}, the decomposition task is hard for algorithms that can be expressed as low-degree polynomials in the tensor entries.
Laura Marcu (BME)
Title: Fluorescence Lifetime Imaging in Surgical Oncology
Abstract: This presentation reviews the development of clinically-compatible fluorescence lifetime imaging (FLIM) technology and applications in surgical oncology. Emphasis is placed on the integration of FLIM in surgical workflow and the potential of this approach to improve surgical decision-making during trans-oral robotic surgery (TORS) and neurosurgical procedures. Clinical outcomes and results will be discussed. We demonstrate the straightforward coupling of FLIM apparatus with the da Vinci surgical platform and the neuronavigation system. Also, we show innovative methods for real-time dynamic augmentation of imaging parameters on the surgical field of view as seen on the da Vinci console and surgical microscope. Current results demonstrate the utility of FLIM-derived parameters detecting tissue biochemical and metabolic characteristics to distinguish oral and oropharyngeal cancer in real-time from surrounding normal tissue in patients in-situ during TORS as well as to sense infiltrative brain cancer at the resection margins. Our findings suggest that label-free FLIM-based tissue assessment, characterized by simple, fast and flexible data acquisition and visualization, could find applications in a variety of surgical procedures.
Xueheng Shi (U. Nebraska-Lincoln, Stat)
Title: Changepoint Analysis in Time Series Data: Past and Present
Abstract: Abrupt structural changes (changepoints) arise in many scenarios, for example, mean/trend shifts in time series, coefficient changes in the regressions. Changepoint analysis plays an important role in modelling and prediction of time series, and has vast applications in finance, climatology, signal processing and so on. This talk reviews prominent algorithms (Binary Segmentation/Wild Binary Segmentation and Pruned Exact Linear Time (PELT)) to detect mean shifts in time series. However, these methods require IID model errors while the time series are often autocorrelated (serial dependence). Changepoint analysis under serial dependence is a well-known difficult problem. We propose a gradient-descent dynamic programming algorithm to find the changepoints in time series data.
This research is joint work with Dr. Gallagher (Clemson University), Dr. Killick (Lancaster University, UK) and Dr. Lund (UCSC).
Stefan Schonsheck (Math)
Title: Representation of Non-Euclidean Domains and Analysis of Signals Thereon
Abstract: Nonlinear dimensionality reduction models have made tremendous impacts on image and signal representation learning. However, almost all extant methods assume that real-valued Euclidean representations are rich enough faithfully preserve the data. However, flat geometry is too simplistic to meaningfully reflect the topological structure of many classes of data with cyclic structures. In the first half of this talk, we develop several methods for accurately representing data sampled from several non-euclidean domains: graphs, manifolds, varifolds, and simplicial complexes. A common theme in this section is to look for approaches that sidestep the curse of dimensionality by defining models that rely on the problem's intrinsic dimension rather than the dimension in which the observation is made. In the second part of this talk, we develop strategies for analyzing signals in these domains, intrinsically solving approximation, regression, and classification problems. This analysis is based on developing generalizations of classical techniques such as multiscale bases and overcomplete transforms, as well as neural network-based machine learning techniques.
James Sharpnack (Amazon)
Title: Lessons in domain adaptation and an unsupervised hunt for gravitational lenses
Abstract: Distribution shift occurs when the training and test distributions differ in a prediction task, and adapting our predictors to this setting is called domain adaptation. In this talk, we will take a tour of the primary domain adaptation methods in classification under the lens of an unprecedented, comprehensive benchmark study and an application to astrophysics. Our benchmark study and accompanying toolkit, RLSbench, consists of 11 vision datasets spanning > 200 distribution shift pairs with varying class proportions. These involve both shifts in image (X) distribution and also label (Y) distribution, which is an often overlooked setting called relaxed label shift. In the second half of this talk, we will apply domain adaptation methods to detecting gravitational lenses in a new astronomical survey (Deep Lens Survey), without the use of known lenses to train with. Instead, we train on simulated lenses and use domain adaptation to generalize to real lenses. We find that with a combination of MixMatch and smart data augmentations, we can dramatically improve model performance (precision at high recall). This study has identified 9 Grade-A lenses, two of which have been spectroscopically confirmed, and 13 Grade-B lenses.